Launch an online presence that makes it simple to attract, win, and serve clients
B12 uses AI and experts to quickly set up your website, scheduling, payments, email marketing, and more.
Get started for free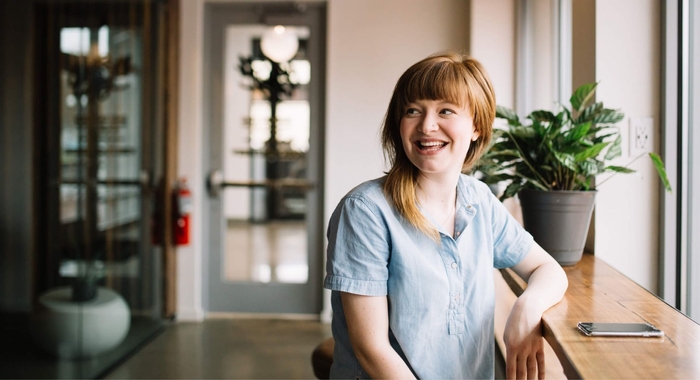
The rising importance of multimodal AI in 2024
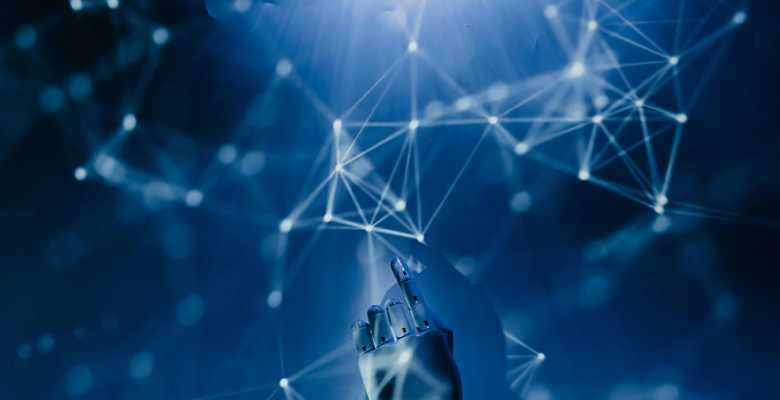
From rudimentary algorithms to sophisticated neural networks capable of powering conversational chatbots and text-to-image generators, AI has transformed into a cognitive force mirroring human thought processes. The integration of these technologies is a significant milestone in AI’s evolution.
Chatbots, with their natural language processing, revolutionized digital interactions. They understand context, providing human-like conversations. Simultaneously, image generators turned AI into a creative force, blurring the lines between artificial and human creativity.
These integrations pave the way for multimodal AI, marking a new chapter in language learning models (LLMs), where collective power surpasses individual capabilities.
The emergence of multimodal AI
Unlike traditional AI models confined to a singular data type, multimodal AI systems are simultaneously designed to process and comprehend information from diverse sources. The ability of an AI model to work with different types of data like text, audio, and images is called multimodality.
Picture a traditional AI system as a specialist focusing on a single task, like understanding text or recognizing images. Now, envision a multimodal AI system as a versatile expert with the ability to seamlessly handle various data types, including text, images, and sound, all at once.
Understanding multimodal AI systems
The key strength of multimodal AI lies in its capacity to integrate different modalities, creating a more holistic understanding of the input data. This integration goes beyond recognizing individual components; it allows the AI system to interpret and respond to complex inputs that involve multiple modes of information.
For example, while a unimodal AI system might excel at reading text, a multimodal counterpart can understand not just the words but also the context when combined with images or sound. This versatility opens doors to various applications, from enhanced user experiences to solving complex real-world problems. According to recent research, the global market for multimodal AI will grow to $4.5 billion by 2028.
How multimodal AI tools work
Multimodal AI systems support a much wider range of tasks than conventional LLMs, providing users with more variety in the type of input they can enter and the output they receive.
You’ve probably come across some popular multimodal systems like Midjourney, Runway, and Dall-E where you enter a text prompt and the output is an image. Some tools like Kaiberr and Neural Frames take text input and convert it into videos and animations. This contrasts a tool like ChatGPT where both inputs and outputs are text-based.
However, in recent years, even ChatGPT has evolved to adopt multimodal capabilities. GPT-4v is a more advanced model with more refined text generation and a better understanding of context.
The capabilities of multimodal AI
What makes multimodal AI stand out is its comprehensive training on an extensive range of media — photos, text, diagrams, charts, sounds, and videos. This diverse training regimen equips these systems with the ability to understand and interpret information from different sources simultaneously.
Incorporating diverse media types in the training process empowers multimodal AI systems to navigate and comprehend complex real-world scenarios.
The second key defining feature of multimodal AI is its ability to generate outputs in various formats, including text, images, and sounds. Unlike traditional unimodal AI models that focus on a single task, these systems transcend limitations and showcase remarkable versatility.
Lastly, multimodal AI systems exhibit proficiency in generating sounds. They not only recognize and interpret audio cues but can also produce sounds in response to various inputs. This capability is instrumental in applications such as virtual assistants and interactive media experiences, enhancing user engagement through dynamic auditory interactions.
Advanced interactions and learning
Multimodal AI allows for expanded functionality in image-based queries. For example, with GPT-4, you could use an image of ingredients as input and ask the tool to suggest food options based on what it identifies in the image. This seamless integration of understanding and generating different media types opens doors to richer, more nuanced interactions.
Another powerful multimodal tool is Google Gemini. This natively multimodal LLM can identify and generate text, images, video, audio, and even code. Gemini excels at spatial reasoning, recently surpassing the capabilities of GPT-4 on 30 out of 32 benchmarks.
This advanced capability enables the AI to not only recognize and interpret but also to creatively respond in different modalities, enhancing user experiences and expanding the range of applications.
Watch: How to use ChatGPT Code Interpreter to create videos from images
Perhaps even more exciting is the realization that things can only get better moving forward. There is general optimism in the industry with Meta’s generative A.I. group Ahmad Al-Dahle expecting the technology to “...get smarter, more useful and do more things.”
What are some real-world applications of multimodal AI?
Several industries are already leveraging multimodal systems to streamline processes and improve decision-making. These include:
- Healthcare — Multimodal AI frameworks have been developed to receive standard electronic health records from multiple input data modalities. This can enhance diagnostic precision and overall patient care.
- Retail — Advanced generative AI allows retailers to create eye-catching marketing images or videos for a brand just by entering a text prompt.
- Customer service — The ability to present information in multiple media formats enhances product recommendations and personalized services.
- Education — Multimodal AI elevates learning experiences with adaptive content and VR/AR devices, catering to different styles.
- Financial services — Next-gen AI strengthens fraud detection by analyzing textual, vocal, and transactional data much quicker than human professionals.
Challenges of multimodal AI
The continuous evolution of multimodal AI promises a future where it becomes more intelligent and versatile, transcending its current capabilities. At the same time, it’s essential to acknowledge its imperfections, reminiscent of similar growth curves with its predecessors — text-only chatbots.
As such, multimodal AI may encounter challenges in accurately interpreting complex scenarios and understanding nuanced contexts. For example, outputs might not precisely align with your expectations and you end up with unprecedented generative fails. Acknowledging these imperfections is crucial, as it lays the foundation for continuous improvement and innovation in the field.
There’s also the tendency for biased output based on tainted input. You’ve probably already come across examples of deep fake technologies being used to perpetrate misinformation and other malicious actions.
Beyond technicalities, it’s also important to account for ethical and privacy concerns, especially when you consider how much sensitive data multimodal AI systems analyze. These include personal images, voice recordings, and location data — details you wouldn't normally share with just anyone.
What’s being done about these challenges?
Amid increased adoption across multiple industries, multimodal AI is set to undergo upgrades aimed at minimizing errors and enhancing human-like reasoning in machine learning.
Advances in generative AI techniques will accelerate the development of the multimodal ecosystem. The demand for analyzing unstructured data in multiple formats is birthing new opportunities at both individual and organizational levels.
The increasing focus on human-machine collaboration will also be a key driver of innovation in this technology. In a recent Deloitte survey, nearly 80% of C-suite executives would support having a chief human+machine resource officer (CHRMO) role in their organizations.
Multimodal AI will be crucial in 2024
Multimodal AI represents a shift from traditional generative AI to more adaptable and intelligent systems capable of processing information from various sources simultaneously and providing output in diverse media formats. This innovation is already revolutionizing industries from healthcare to finance and will likely be a key driver for AI advancement in 2024 and in the years to come.
Look professional online with tips from B12
Receive our email newsletter for advice on how to grow your business and engage clients.
Spend less time on your website and more time growing your business
Let B12 set up your professional online presence with everything you need to attract, win, and serve clients.